New antibody tests might be game-changers in keeping the public safe from infection while restarting the economy. Giving “immunity passports” to those who have tested positive for having had the disease would allow thousands of people to return to work.
However, there is as much controversy as there is excitement about this new idea. Foremost in many people’s minds are the ethical implications. People in difficult economic circumstances may, perversely, be incentivised to contract the disease so they can return to work. For others, privacy issues related to centralised storage of medical data is a stumbling block. The World Health Organisation has also cast doubt on the extent to which people who have recovered from Covid-19 will be protected from future infection.
Perhaps the least well understood of the concerns is the accuracy of the tests. The United States Food and Drug Administration has granted an emergency use authorisation to seven manufacturers to bring antibody tests for Covid-19 to market.
One of the first tests to gain authorisation was developed by Cellex. If you have antibodies against Covid-19, their test will tell you this correctly 93.8% of the time. This is the test’s “sensitivity”. If you don’t, it will get this correct 95.6% of the time. This is the test’s “specificity”. Getting the correct result more than 90% of the time sounds pretty encouraging.
But let’s consider what would happen if the test were given to 10,000 people as in the diagram below. Although estimates vary significantly, the World Health Organisation suggested recently that as few as 3% of the global population may have had Covid-19 and recovered. This means that 9,700 of the 10,000 tested will not have had the disease and only 300 will have. Of the 300 recovered patients, 93.8% – or 281 – will be correctly told they have antibodies against the disease. Of the vast majority, 9,700 people who haven’t had the disease, 4.4% – or 427 – will be incorrectly told that they have had the disease and recovered.
In short, far more people will receive false-positive results than true-positive results. Up to 60% of those released back into the workforce could be at risk of infection themselves and unknowingly spreading the disease to others, sparking a second wave of the epidemic. If the true prevalence of the disease in the population is as low as 1% then this figure could rise to 80%.
The problem of false positives outweighing true positives occurs in any situation for which the prevalence of a disease in the tested population is low and the test gives a significant proportion of false positives. As I uncover in the Maths of Life and Death, this situation is common in screening programmes. In breast cancer screening, for example, false positives can outweigh true positives by a ratio of three to one, leading to significant anxiety and the potential for unnecessary procedures.
Double testing not full proof
Repeating the same antibody test could reduce the rate of false positives, though. Retesting those people who tested positive on the first test and issuing immunity passports only to those who have received two positive results could reduce the proportion of false positives to below 7% – see diagram below – a significant improvement.
But double-testing only works if the results of the two tests are independent. If, however, the reason for the false positives is systematic – detecting antibodies from other coronaviruses, for example, then there is no reason to believe a second test will do any better than the first.
False negatives
While false positives are a problem in the wider community, hospitals can face an acute problem as a result of false negatives. For a variety of reasons – including inaccurate swabbing and variable viral load – the RT-PCR test used to diagnose people who currently have Covid-19 gives a false-negative rate of up to 30%. In the mirror image of the situation in the wider community, when the prevalence of a disease in a group is high – as in those admitted to hospitals with suspected Covid-19 – false negatives swamp true negatives with potentially disastrous consequences.
It’s natural to assume that people going to hospital with severe symptoms of Covid-19 probably have the disease. These people must be correctly diagnosed so they can be isolated from the general hospital population and treated.
Assuming that 90% of these cases will have the disease, it’s natural to ask what proportion of negative test results are correct. Using the same mathematical argument as before, considering a representative sample of 10,000 patients, the diagram below shows that, in this setting, a negative result might be correct as seldom as one case in every four.
This is a huge problem for hospitals. Patients who should be being isolated may be incorrectly sent to Covid-19-negative wards and receive inappropriate treatment or even be sent home thinking they are not infectious only to spread the disease widely.
Understanding the startling rates of false positives and false negatives for tests that seem, on the surface, to be quite accurate could have profound consequences for health policy as we travel deeper into this pandemic. Failing to do our mathematical due diligence has the potential to take us past the tipping point beyond which the epidemic starts to grow again, leading to even more avoidable deaths.
Christian Yates, Senior Lecturer in Mathematical Biology, University of Bath.
This article first appeared on The Conversation.
Buy an annual Scroll Membership to support independent journalism and get special benefits.
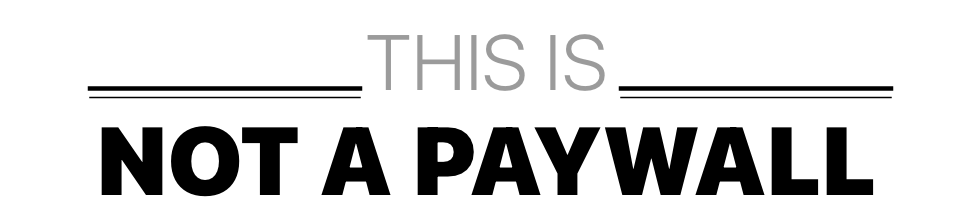
Our journalism is for everyone. But you can get special privileges by buying an annual Scroll Membership. Sign up today!