Various models are being proposed to bring us out of this lockdown. Although, as Harvard’s Ashish Jha has forcefully argued, it might be that we know too little about the status of Covid-19 in our populations to make that decision just yet, it is now clear that part of the strategy is a move from a general to a “graded” lockdown.
This graded system punishes states if they are judged to be insufficiently compliant with the lockdown rules, and uses a threshold Covid-19 prevalence to stop all movement at the level of the district. Apart from the fact that states will now have a disincentive to report cases, this strategy also raises the question of whether there are other, perhaps more nuanced approaches that can be used to create finer categories.
One idea that has been proposed is that of risk stratification: hospitalisation risks for Covid-19 increase with age and pre-existing chronic conditions. Therefore, allowing for greater movement among the young and healthy while protecting our elders will allow for the resumption of economic activity and gradually build up immunity in our populations, even though the young will remain more likely to be infected.
Locking down smaller areas
Like the color coding of districts according to current Covid-19 infections, risk stratification can be spatially targeted by maintaining stricter restrictions in places with a larger agglomeration of older people and/or those with additional co-morbidities. One particular advantage of spatially targeted risk stratification is that we can think of smaller units in terms of lockdown grades. For instance, rather than locking down a district, we can lockdown villages or even urban neighborhoods that are at higher risk.
Whether this can be accomplished in practice depends on the underlying degree of variation. Do we really have some villages and urban neighborhoods with an agglomeration of Covid-19 risks?
A simple back-of-the-envelope computation shows that we might. Consider age, which has been a major factor in determining the number of hospitalisations. According to 2016 census estimates, 5.4% of India’s population is above the age 65, and if we assume that the fraction is the same in rural and urban areas, this gives us around 45 million people above the age of 65 living in 641,000 villages, for an average of 70 per village.
There will be a large variance, so that some villages may have as few as 10 people above the age of 65, while others will have multiple times that number. One approach could therefore impose stricter conditions in villages with a large elderly population compared to villages where the number of the elderly is small.
What about urban areas? And what about other co-morbidities that we know will affect the risk of mortality conditional on hospitalisation?
As a first attempt to examine this question, we used data from the fourth National Family Health Survey to construct a profile of 324 clusters in Delhi in terms of the risk of hospitalisation conditional on falling sick. These data are far from ideal – we do not know the precise location of the approximately 21,000 adults (aged 15 and up) in this survey; the data on hypertension and diabetes are based on a further sub-sample, and the cluster locations themselves are “jittered” to preserve individual anonymity in the database. We also do not adjust for the number of children as youth infection dynamics are highly uncertain.
Nevertheless, the exercise is useful because these imperfections in the data should actually make neighborhoods look more alike than they really are. Therefore, if we find substantial differences across clusters in these data, in “real life” the differences should be even larger.
To construct cluster-level and household-level risk profiles, we used a simple index that combines age, sex, smoking status, and hypertension. Specifically, our index gives 2 points to every person aged 65 or older; 1 point each to being male, a smoker, or having hypertension and 0.5 points to having diabetes. For example, a young woman with none of these characteristics would have a score of zero; a 70-year-old man who smokes and has hypertension and diabetes would score 5.5.
This is somewhat arbitrary since the literature to date does not allow for more precise weighting of these factors, but (a) it is consistent with the literature on mortality risks (conditional on hospitalisation); (b) can be modified easily once more information becomes available (it is one line of code), and (c) at least allows us to check if there is variation in these characteristics across neighborhoods.
The figures above andbelow summarise our findings. In the first figure, we have shown a histogram of neighborhood riskiness according to this crude metric, where green is least risky and red is highly risky (split roughly into 25% of the neighborhoods each). There are a large number of neighborhoods in Delhi that are green, but also a longish tail that is red; it is the red areas where we would expect the highest number of hospitalisations to come from if Covid-19 hits.
To give some idea of what these colours mean, in green neighborhoods, out of every 100 people, the total number of risks varies from 50 to 65, while in red neighborhoods it ranges from 100 to 134. Most of the variation is due to age. Similar to the Indian census figures, only 5% of the population in the National Family Health Survey sample is above the age of 65. This is a combination of life expectancy and that fact that in-migrants tend to be young, with older populations disproportionately located in certain areas.
An interesting contrast is among the Trans-Yamuna neighborhoods: areas like Naveen Shahadra, Patparganj and Vasundhara Enclave have a more risky profile, but northern areas like Dilshad Gardens all the way to the Loni border tend to have younger populations. This seems reasonable; the former areas have become home to many people who have retired from their jobs and brought apartments in these neighborhoods, while the latter are home to migrants from neighboring villages and therefore predominantly young. To protect populations and capacity in our hospitals, it may make sense to enforce greater restrictions in the former.
In these data, the number of risks per person are similar across more and less wealthy households although the reasons are different: Richer households have more people who are old, and more people with hypertension. Poorer households tend to be more predominantly male and with higher rates of smoking. This is a more hopeful scenario, as economic crisis will be (and already is) most severe among the poor neighborhoods. Using these crude measures of risk stratification suggests that the economics and the public health criteria may move in the same direction; the impacts of graded lockdowns would be substantially worse if risk profiles were also the worst in the poorest areas.
Three ways to augment the approach
This, however, is based only on the risk of mortality conditional on hospitalisation and does not account for the potential difference in risk of infectivity. Moving forward, such spatial targeting approaches can be augmented in three ways.
First, pre-existing information on age, sex and co-morbidities at a granular geographic level can be combined with state-of-the art methods from small area estimation to construct hospitalisation risks.
Second, similar exercises could be conducted for risk of infection (which will also depend on, for instance, population density and movement) and the risk of hospitalisation (which could depend on other co-morbidities in populations such as ours – we don’t know, for instance, how long-term exposure to pollution will affect these risks).
Third, better data on who is being infected, who is being hospitalised and who is ultimately dying would also allow for rapid, real-time updating of such models.
As the costs of shutting down the economy start to mount, it is clear that we need to move from total lockdown to smart containment. Spatially targeted risk stratification may present one way out of this dilemma.
Jishnu Das is a visiting member of faculty at Centre for Policy Research and a professor at Georgetown University.
Benjamin Daniels is a Research Fellow at Georgetown University.
Scroll has produced award-winning journalism despite violent threats, falling ad revenues and rising costs. Support our work. Become a member today.
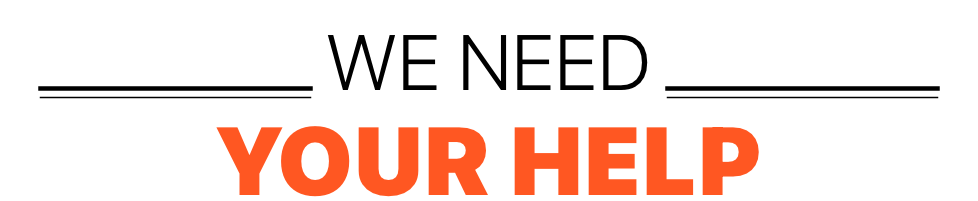
In these volatile times, Scroll remains steadfastly courageous, nuanced and comprehensive. Become a Scroll Member and support our award-winning reportage, commentary and culture writing.